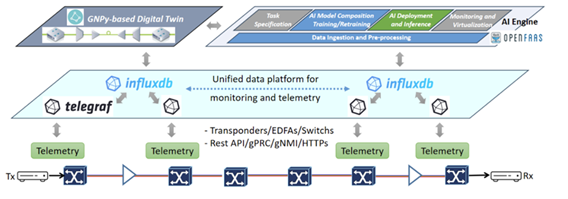
Optical Link Performance Monitoring Experiments
A GNPy-based digital twin and unified monitoring platform were developed, combining AI, telemetry, and real-time data processing to achieve highly accurate QoT predictions and revolutionize dynamic optical network management, validated through a 493-km field-trial testbed.
A GNPy-based digital twin was developed to enhance end-to-end Quality of Transmission (QoT) prediction by seamlessly integrating network telemetry, time-series databases, and an AI engine, all tested over a 493-km field-trial testbed implemented on NDFF. This innovative digital twin generated reliable synthetic data critical for model pre-training. By leveraging transfer learning, our QoT prediction models achieved significant accuracy improvements, with prediction errors limited to just 0.25 dB across multiple links. This breakthrough demonstrates the potential of digital twins for dynamic optical networks, setting a new benchmark for end-to-end QoT prediction.
To complement this, we developed a unified monitoring and telemetry framework featuring a Kafka-based telemetry pipeline, advanced machine learning (ML) applications, and time-series data storage via InfluxDB with multiple functional plugins. This robust platform ensures real-time data collection, processing, and streaming with a latency of less than 0.05 seconds. By combining advanced telemetry with ML technologies, the platform enables proactive network management and informed decision-making with unparalleled performance, reliability, and operational efficiency.
For real-world validation, we conducted a three-week optical monitoring experiment on the NDFF field-trial testbed to assess short-term QoT prediction. The unified monitoring and telemetry platform was also deployed in this real-world setting, demonstrating its practical applicability and highlighting its capability to revolutionize dynamic optical network operations.
Fig. 1: The schematic view of the GNPy-based digital twin with network telemetry
Funding information: This work has been funded by European Union’s Horizon RIA project ALLEGRO (No.101092766)
For further information: contact Dr. Rui Wang, ndff@ee.ucl.ac.uk
Published: 27 February 2025