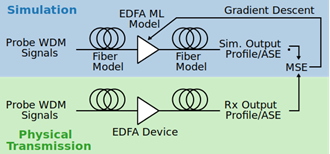
CASE STUDY: Spectral power profile optimisation of WDM transmission system by remote link modelling
Machine-learning experts from the Technical University of Denmark (DTU) partnered with the NDFF team at the University of Southampton for an experiment that optimised a WDM fibre network implemented on NDFF, using a model of an erbium-doped fibre amplifier obtained through training performed from a single access point.
The overall goal of this work was to develop models that predict the signal power and the accumulated noise power for all WDM channels of a link as a function of its input power profile. These models can then be used to optimize for operation-specific properties of the link via gradient descent. The model comprised of analytical fibre models and trained erbium-doped fibre amplifier (EDFA) machine-learning (ML) models in cascade. The EDFA model predicts the amplifier gain profile and the profile of the amplified spontaneous emission (ASE) added by the amplifier as a function of the input power profile and the amplifier operating point (its total input and total output power). The modelling methodology is outlined in Fig. 1.
Fig.1: Remote modelling of EDFA on NDFF
To model the EDFA at the remote colocation site (Reading), the physical model weights of the amplifier were initialized, and a cascade digital twin model of the fibre-EDFA-fibre link for the Southampton-Reading-Southampton part of NDFF was created. The obtained EDFA ML model was used in cascade with fibre models and a transmitter penalty model for performance prediction and gradient descent optimization of the input power profile to chosen path configurations on the network. A cost function was defined targeting a flat SNR.
Fig. 2: Path configurations considered for the transmission experiments.
For the transmission experiments, two different path configurations on NDFF were considered (Fig.2). The transmitter (at Southampton – STN) emulated a 48 channel WDM system with a channel spacing of 100 GHz. A setup of EDFAs and WSSs emulated 47 WDM channels from an ASE noise source of bandwidth 12.5 GHz, and a single data channel was loaded with a modulated signal, which was multiplexed into the system on the wavelength under test. The data channel used polarisation multiplexed 64QAM with probabilistic amplitude shaping (PAS) at a baud rate of 15.33 GBd. The WSSs were further used to apply an arbitrary power profile across the WDM channels which were launched into the network.
Four nodes of NDFF were employed in the experiments (as shown in Fig.2), with 6 EDFAs in total - 4 in RDG and 2 in PGT. The two path configurations used were STN-RDG*-PGT*-RDG*-FRX-RDG*-STN (a * denotes an amplification node) of a length of 439.4km, and STN-RDG*-PGT*-RDG*-PGT*-RDG*-FRX-RDG*-STN, of a longer length of 592.4 km.
Fig. 3: SNR decoding threshold for the two link lengths before and after optimisation.
The received SNR of up to 24 out of the 48 WDM channels was measured. The SNR margins were evaluated for the cases when a flat spectral profile at the input and one that was optimized using the ML-based model. The minimum margin was found to improve from ≈ 0.8 dB to ≈ 3 dB and <0.1 dB to ≈ 4.8 dB, for the long and short link, respectively. Furthermore, the improvement of the margin in the long wavelength region was ≈ 2.2 dB and ≈ 0.8 dB for the long and short link, respectively.
Funding information: This work has been funded by EP/S028854/1, EP/S002871/1 and Innovation Fund Denmark project RA-NON, ref. 1047-00013.
Further information: https://arxiv.org/abs/2207.01336
Contact: Professor Periklis Petropoulos, ndff@ee.ucl.ac.uk
Published: 06 March 2023